Artificial Intelligence is an indispensable part of the finance world due to its ability to understand complex mathematical relationships and its automation ability. With data and compute power growing exponentially, financial service providers turn to AI for cost-effective solutions.
A Brief History of AI in Finance
Though Artificial Intelligence has become a buzzword, it has always existed in some form for quite some time. We can trace the origin of AI to classical philosophers’ times when they tried to equate human thinking to a symbolic system. However, AI never received official recognition until the 1950s. In 1956, the term “artificial intelligence” was coined at the Dartmouth Summer Research Project on Artificial Intelligence (DSRPAI) hosted by John McCarthy and Marvin Minsky1. Though many disagreed on standard methods, overall, the conference saw an encouraging response.
There was a massive growth in paperwork after World War 2, which created a growing need for systems to automate some of the work. However, researchers also estimated the risk of job losses due to automation.
In the 1960s, research focused on Bayesian statistics and was used heavily in machine learning2. The application of Bayesian Theory in the auditing area is the reason behind its popularity. While auditing, the auditor’s judgment is based on professional expertise. However, different situations and various sudden developments call for different approaches to decision-making. The Bayesian model offers objective and rational probability to empower auditors to make more accurate assessments. It also eliminates misjudgment caused by the personal emotion of the auditor.
Experts welcomed the innovative technology, and AI flourished until the 1970s.
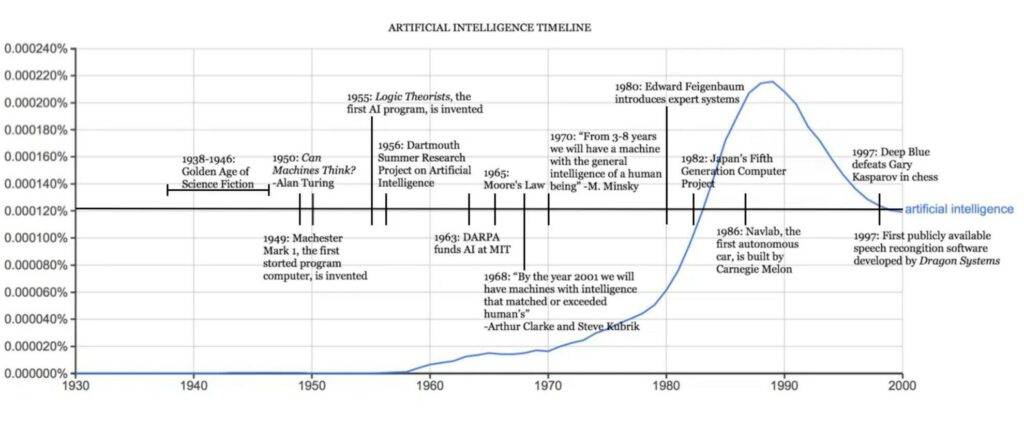
It hasn’t always been a smooth ride for Artificial Intelligence. Several reports were criticizing the developments in AI. The mid-1970s to 1980 was known as the “AI winter,” when the government funding and interest in the field had died down. The buzz surrounding AI only resumed in 1983 when the British government’s research project, Alvey, was funded from the war chest of GBP 350 million to compete with the Japanese Fifth Generation Project.3
According to Andrew Ng, a veteran computer scientist, neural networks made their presence felt in the 70s and gained prominence in the 80s, after which they fizzled out. However, today, there is renewed interest due to the availability of vast amounts of structured and unstructured data and the proliferation of cost-effective compute power.
In the 1980s, more than two-thirds of Fortune 1000 companies had at least one AI project under development.4
In 1982, AI made its presence felt in the financial services industry when James Simons, an award-winning mathematician, founded quantitative hedge-fund Renaissance Technologies.5 As of April 2021, Renaissance had a market capitalization of $165 billion.6 The firm leverages data in its petabyte-scale data warehouse to analyze statistical probabilities for the trend in securities prices in any market. These mathematical models are based on massive data analysis, then analyzing non-random movements for making predictions.
The Launch of Expert Systems
The 1980s was when AI began to gain prominence in the finance world. This was when Expert systems emerged as an excellent commercial product in finance. Until the 1980s, the Expert System (intelligence system based on knowledge) was used in the financial industry to predict market trends and provide customized financial plans.
The banking industry and financial institutions began to increasingly use expert systems to reduce the risks of human mistakes. It helped in financial analysis, market analysis, business development, international business, currency exchange, and bank management. Edward Feigenbaum introduced expert systems in 1980, which emulated the decision-making expertise of a human.7
Dupont built 100 expert systems which helped them save nearly $10 million a year.8 The Protrader expert system was designed by KC. Chen and Ting-peng Lian were among the first expert systems that forewarned the 87-point drop in DJIA in 1986.
At the same time, Applied Expert Systems (APEX), called the PlanPowerOne, was the first expert system that helped with financial plans. It was first commercially shipped in 1986, and it helped create financial plans for people with an annual income over $75,000. Similarly, a Client Profiling System designed financial goals for $25,000 and $200,000 a year.8
Cut to the 1990s, when the hype was more about fraud detection. The FinCEN Artificial Intelligence system (FAIS) reviewed over 200,000 transactions per week. Over two years, the system could identify 400 potential money laundering cases worth nearly $1 billion.8
In 2003, Hodgkinson and Walker developed a rule-based Expert System to help financial intuitions make underwriting decisions on the credit application of corporate loans.9
In 2009, LY Shue, CW Chen, and W Shiue established an Expert System that contains the domain and operational knowledge base to conduct an ontology-based ranking of listed companies in the Taiwan stock market.10
Janulevicius and Goranin, 2013, built up a risk management Expert System to enable medium and small-sized enterprises to conduct professional data security analysis due to capital constraints.10
Expert systems aren’t a part of the finance sector anymore. However, it has been instrumental in jump-starting AI and introducing its application in finance in the modern world.
Impact of Natural Language Processing
The financial world massively depends on numeric data to process critical information. However, the dependence isn’t just limited to numeric or quantifiable data alone. A massive amount of text is also involved that waits to be analyzed for deeper insights. This is where Natural Language Processing (NLP) plays a crucial role in the financial sector. NLP is a subset of Artificial Intelligence (AI), wherein computers can read and interpret words and sentences just like humans.
NLP has transformed the finance industry through accurate and efficient deciphering of text. The innovative technology finds many applications in the compliance and back-office divisions, customer service experience, and portfolio management. NLP can parse complex audio and textual content related to business and finance. These include transcripts, sec filings, earnings releases, press releases, and sector reports that contain a lot of industry jargon, currencies, numbers, and product names.
In 2017, State Street Corporation launched Quantextual Idea Lab, which uses NLP and machine learning to efficiently organize and extract information from various types of content.24
To understand the use of Natural Language Processing in financial services, take a look at this video. Leading global chipmaker NVIDIA opens up about how the industry will use conversational AI in finance for the better:
How is AI Used in Finance?
Artificial Intelligence has been a matter of interest for academicians and science-fiction writers. However, there has been an unprecedented rise in the buzz around AI in recent years. This innovative technology has become widely adopted for real-world applications, particularly in business and finance. This is primarily due to three reasons:
- The abundance of data: The volume of structured and unstructured data produced daily is enormous. According to IDC, 64. Twenty-two zettabytes of data were produced or replicated in 2020/data is the fuel for new algorithms to predict the output.11
- Compute power: Processing power is now cheap enough to solve many of the previously cost-prohibitive problems.
- Complex algorithms: Improvements with artificial neural networks have occurred at breakneck speed.
The survey, which studied responses from 151 financial institutions from over 30 countries, confirmed that AI is a critical business driver across the industry.12 64% of the financial institutions expect to become mass adopters of AI in the next two years, aiming at its potential to drive innovation and growth across a wide range of finance-related functions.12
Talking only of Fintech, the use of AI is likely to grow by more than $22.6 billion by 2025. 13 The widespread demand for process automation among financial services providers is a decisive factor driving the growth of AI in the Fintech sector.
Cognitive process automation, an evolved form of Process automation, allows systems to perform highly complex processes by replicating human Intelligence. However, a survey by Finrail revealed that 33% of Fintechs in 2019 used AI solutions that they have developed in-house.14 Thus, there is a preference for in-house software over purchasing it from third-party vendors.
Another joint research report by National Business Research Institute and Narrative Science stated that 32% of financial service providers use AI technologies such as Voice Recognition and Predictive Analytics.15
AI brings a plethora of innovative solutions that ease the task of finance and accounting professionals. Machine Learning, Natural Language Processing, and interactive chatbots have altered the operational landscape of finance professionals. AI-powered technology is gradually taking over specialized duties like decision-making, financial modeling, and financial analysis, not just the repetitive and mundane tasks.
Financial Services Sector Representation
The pie chart below illustrates a survey conducted on the Fintech respondents. The companies revealed that 40% of the time, they use AI for managing deposits and lending activities, while 25% of the time, AI is used for marketing activities.
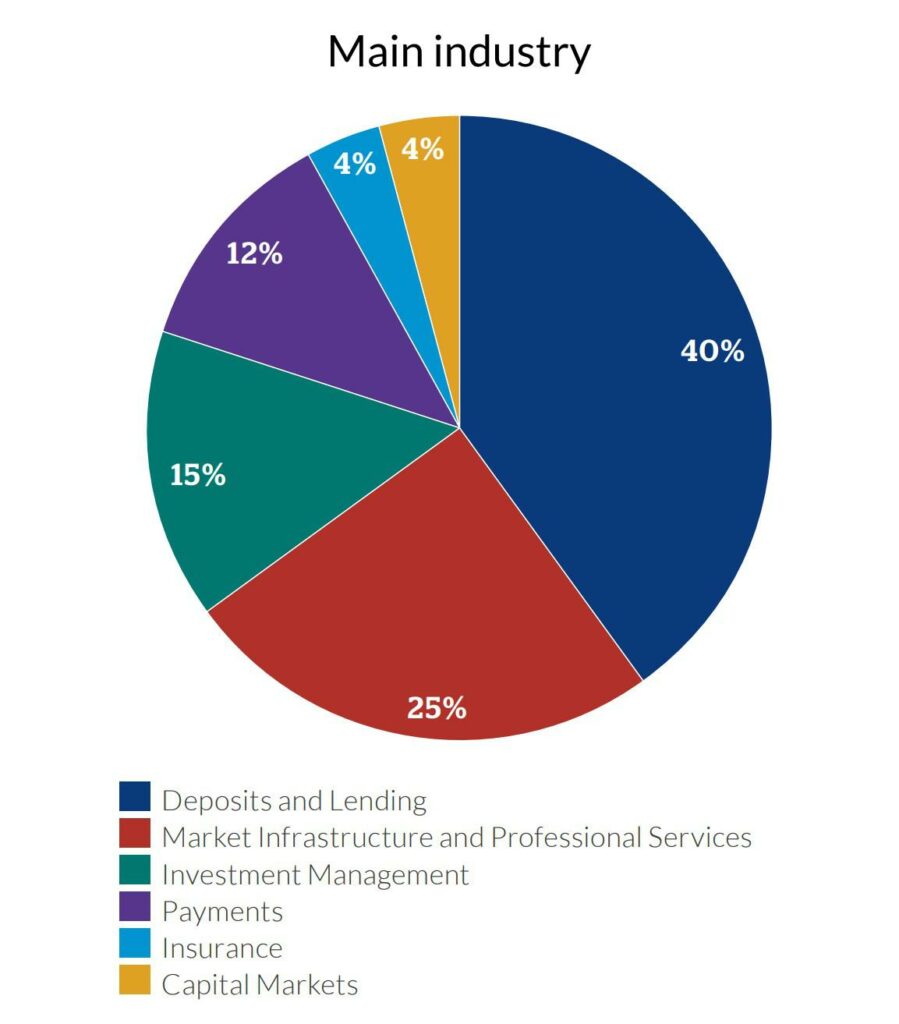
When I talk about AI applications in finance, I don’t want to restrict the discussion to financial institutions or the banking industry. Even the FP&A and F&A verticals of companies from other industries have begun to adopt AI for various business functions.
Tax professionals, who need specialized skills to stay abreast with a nation’s regulatory requirements, are also increasingly using AI for finance. AI can transform the required data and make it more credible.
According to PwC, Microsoft uses machine learning and intelligent process automation (IPA) in analytics that performs real-time sales reviews to ensure resellers’ compliance with the Foreign Corrupt Practices Act. Citigroup developed robotic process automation (RPA), NLP tools, and data visualization to automate processes traditionally performed by business analysts, such as financial reporting and generating management commentary on financial results.
Take a look at how J.P. Morgan, a leader in financial services, implements AI into its daily operations:
AI is helping the financial industry to optimize and streamline complex processes, including credit decisions, quantitative trading, and risk-based management.
Here’s a brief rundown of all the areas in finance that AI is gradually transforming:
Loan Underwriting
Owning a credit card has become increasingly common today. According to a study, 77% of consumers favored making payments through a debit or credit card, while only 12% preferred cash.16 Besides convenience and easy availability, a good credit record helps secure finances of any kind. AI solutions aid credit lenders and retail banks to make smarter sanctioning decisions. This is done by leveraging various metrics to assess the borrower’s credit risk and credit history more accurately and quickly in the loan underwriting process.
Credit Scoring and Churn Predictions
Most of the credit scoring systems used currently are redundant. They base their decisions on estimating a customer base, demographics, marital status, etc. However, the advanced scoring systems target real customers and use the data science-powered churn prediction software to study the possible outcomes. The churn prediction analyzes each loan application and can cap the quantum of customers lost to 45%.
The algorithms analyze historical user data points through the financial behavior of the applicants, previous loan applications, marital status, debt situation, etc. According to Dmitry Dolgorukov, CEO of GiniMachine, an AI-based credit scoring platform, such systems can lower Non Performing loans by up to 53% and increase revenue by 37%.17 From a marketing perspective, they can also design the perfect campaigns by targeting the right customers and reducing churn risk.
Claims Management and Fraud Detection
Using a combination of AI and ML, insurers can gain multiple benefits. Advanced Machine Learning techniques can handle different phases of the claim management system, and Artificial Intelligence can help insurers automate the process. These systems help quicken the claim settlement, reduce the processing time, enhance customer service, and help lower handling and operational costs. During the process, AI tools monitor the user’s behavior and identify fraudulent transactions. The self-learning abilities of AI systems can then adapt to newer cases, enhance detection power over time, and implement anti-money laundering practices.
Let’s take Plaid, for instance. It works as a widget that connects a bank with the client’s app and secures financial transactions.18 Its complex algorithms can assess interactions under different scenarios and variables and build multiple unique patterns updated on a real-time basis.
Risk is a part of any business, and it is something that you cannot ignore. If proper steps are not taken correctly, the inherent risks can snowball into issues too big to handle. However, companies can nip predictable risks through accurate and timely detection and forecast.
Besides existing risks, additional risks can evolve with the situation and the company’s growth cycle. For such cases, the corporate sector’s financial institutions or finance divisions must have an agile system to react to sudden changes. That’s why the financial industry is utilizing more and more Machine Learning to design elegant models. The algorithms used in risk management help companies analyze and identify trends and perform effective future planning.
Quantitative Trading
Quantitative trading involves using massive data sets to identify patterns and make strategic trades. This is one such area of trading where Artificial Intelligence is beneficial. AI-powered computers can analyze vast and complex data sets quickly and efficiently. The resulting algorithmic trading processes automate trades and save valuable time.
AI has been used in financial platforms to utilize Natural Language Processing to assess keyword-related searches in transcripts, research, filings, news articles, etc., to decode the underlying trends in the financial markets. Machine Learning and quantitative analysis process humongous data sets and identify real-life patterns in the financial markets. The algorithms study complex and vast data and condense the raw data into meaningful pieces of information.
In addition, due to its high forecasting power, Artificial Intelligence is widely used in short-term stock price prediction for Algo trading.
Customized Banking
The world is going cashless and digital payments have become the order of the day. As businesses and individuals adapted to the new normal, online modes of payment grew at an unprecedented pace during the pandemic. There has been a sea change in the process of banking, and the traditional way isn’t striking a chord with the digitally-inclined customers anymore.
A survey by Accenture revealed that 54% of the customers wanted tools for monitoring their budget and making real-time spending decisions.19 Not just this, 41% of the customers are also interested in receiving guidance from banking chatbots.19 These chatbots use Artificial Intelligence to offer personalized financial advice, while natural language processing provides instant customer service.
Cybersecurity Protection
Cyberattacks are getting more sophisticated by the day; hence it is imperative to set up a detection system that is a notch higher than the average. Experts suggest that about 95% of cyberattacks and data lapses happen due to human errors, and AI has conquered almost every financial service sphere of all these problems.
The AI system allows you to detect loopholes in the security system and find solutions through complete data analysis within milliseconds. In addition, the analytical capacities of machine learning software to detect complex patterns in ways that can be highly challenging for a fraud analyst to see.
Predictive Analysis
Predictive analytics directly impact overall business strategy, resource optimization, sales promotion, and revenue generation in financial services. Predictive analytics uses vast amounts of data to detect patterns and predict insights.
These results and insights can help financial service providers to reveal the next course of action. Predictive analytics include everything from sophisticated statistics to Data mining. It can help CFOs use historical data and identify trends for precise planning, forecasting, and decision-making. Using ai capabilities, the finance functions can predict outcomes, identify new opportunities, expose latent risks, and anticipate the future more accurately.
Why is AI the Future of Finance?
According to Accenture, AI and augmented technologies are likely to contribute $140 billion in productivity gains and cost savings to the financial services companies globally by 2025.20 Global financial services are at the cusp of a radical change in the future. Insurance companies and many banks believe that AI can unlock new growth opportunities and lower operational costs.
A survey by the Economist revealed that investment banks had been the frontrunner in terms of AI adoption. Due to their massive size, investment banks deal with several complex, large-scale challenges. Implementing innovative technology such as AI can offer critical solutions to these problems.
Advanced technologies like machine learning, predictive analysis, and natural language processing have taken over finance in a big way. Not just investment banks, even retail banks, are leading in adopting these highly transformative technologies. Automating manual tasks will not only lead to cost control but will also streamline the workforce.
Digital assistants and apps will continue to get better with enhancements in cognitive computing, such as deep learning. With routine tasks getting automated, consumers and employees in the financial services sector are more likely to focus on what matters, such as formulating strategies and longer-term decision-making.
Pros and Cons of Using AI
AI Benefits
AI applications in finance can lead to enormous benefits. Artificial Intelligence in finance can help automate a lot of work, but it can also help identity theft and improve customer experience. A lot of anti-money laundering initiatives will also be conducted through Artificial Intelligence. According to a report by Accenture, “AI will enhance the value of the financial industry by $1.2 trillion by 2035.21
Before we discuss the holistic market condition, here’s a quick look at the significant advantages of adopting AI:
Efficiency and Speed
Artificial Intelligence is efficient in handling large volumes of information. Soon we could have real-time dashboards available in an instance. These metrics will be beneficial for FP&A professionals and increase efficiency in budgeting and forecasting.
In addition, when professionals use these insights and metrics, they will have more time to build business relationships and conduct high-level beneficial advisory work.
Error Reduction
AI-based applications can lower the level of human errors drastically. Computer algorithms are far more efficient and do not get bogged down by repetitive calculations. As a result, the degree of mistakes is far limited.
Indeed, AI cannot replace human Intelligence. Still, companies can use it to automate mundane tasks and calculations, giving human intelligence scope to listen to high-level issues that demand creativity and unique problem-solving capabilities. Reports reveal that AI has an accuracy level close to 95%. However, there is a catch here.
Better Data Storytelling
As data becomes an integral part of organizations, each stakeholder must know how to communicate the insights from data effectively. Artificial Intelligence enables algorithms to understand and respond to information just like humans or even better.
Through a process called Data visualization, algorithms create data-driven imagery to allow people to decipher the details in a better way. Storytelling with data empowers businesses to utilize crucial metrics by presenting compelling stories and transforming them into actionable insights. Data storytelling helps analyze key performance indicators (KPIs) that can convert quantitative data into result-oriented information.
Cost Reduction
Traditional financial institutions can use Artificial Intelligence to achieve nearly 22% or $1 trillion in projected cost reduction by 2030.22 AI brings numerous benefits and results in high ROI. Artificial Intelligence helps businesses to target customers effectively.
Through sales intelligence, companies can analyze a particular buyer’s purchase behavior with increased precision. Leveraging Artificial Intelligence in key strategies allows a business to conduct its operations seamlessly. Companies can create More transparency while reducing the cost of complex business practices.
AI Drawbacks
Technology “evangelists” excel at creating the buzz around artificial Intelligence by focusing on its promises. However, reaping the benefits from intelligent algorithms can be very challenging in the real world.
Data Quantity & Quality
The quality and quantity of input data dramatically influence the accuracy of the prediction power of an algorithm. Even if data is taken from credible sources, there may be hidden biases. Moreover, we cannot rule out the amount of time taken to clear the data of anomalies. The reconciliation of the data from the front to back end in the financial industry is very cumbersome. Moreover, the data suffer from quality issues.
Hence, for an artificial intelligence unit to function efficiently, it must have a sacrosanct data-quality program. Finding and organizing data properly is one of the biggest challenges for ai in finance and other industries.
Black-Box Effect
The calculations of the artificial intelligence algorithms are not transparent. The statistical results they deliver could be wrong if compared on a case-to-case basis due to hidden biases and anomalies. It is a complex process to identify these biases and correct them. It is also very challenging for a banking or financial institution to explain each Artificial Intelligence algorithm’s outcome. The Artificial Intelligence black box exposes the organization to economic and ethical risks.
Thus the FP&A teams need to have clear foresight, anticipate the upcoming issues and challenges and prevent unethical applications of the emerging technologies. One of the biggest challenges I struggle with when creating reinforcement learning trading algorithms is this. It’s challenging to get behind an algorithmic trading strategy when you’re unsure how it works!
Lack of Accountability
The use of Artificial Intelligence demands a lot of responsibility. Financial institutions are still yet to give full autonomy to machines and algorithms because their behavior is unpredictable.
As a result, the incorrect have to spend resources to appoint a human supervisor to monitor and validate the machine’s decisions for crucial activities. These include highly critical actions like managing payments and validating trades. This partially defeats the purpose of employing a device at the outset.
Lack of Safety and Regulation
If data is misused in the fintech business, there could be massive losses. Therefore constant improvements in security procedures are critical for adopting AI. AI is evolving and entering new industries at a lightning pace. This makes it increasingly challenging for the companies and the governing bodies to regulate them.
Interestingly, the most significant advantage of AI becomes the biggest challenge for regulation. When a machine is performing the activity, there is no accountability. If any mishap occurs with driverless cars, a stock market crash happens due to algo trading, or a credit analysis goes significantly wrong due to automated programs, who is liable? The lack of clear regulatory policies about AI is a significant hindrance to its adoption.
Limited Function Scope
Even the most intelligent algorithms are designed to solve particular problems, and there can be no deviation in the way they are designed to work. Algorithms are backed by pure logic, but they lack contextual thinking ability and emotional Intelligence like human beings.
A program meant to identify payment fraud will only do that. It is so restrictive that it may fail to detect any other suspicious activity related to payment and transaction. Algorithms are intelligent, but they also lack empathy. Chatbots are the most prominent example of this. They may have all the answers to your queries, but they cannot empathize with or understand your concerns. Connecting with customers more emotionally is a requisite for B2C companies.
In my opinion and experience researching AI, we’re still far from general Intelligence.
Shortage of Skilled Professionals
One of the most significant issues impacting the AI industry today is the limited supply of AI and ML data scientists. The specialists in the field are limited, which could lead to a massive resource backlog.
The specialized technical institutions and the financial sector must take enough initiatives and offer incentives to boost participation in this field, but the desired progress in AI will be stalled.
How Will AI Transform the Financial Industry?
The applications of AI in finance are indeed redesigning the business landscape of the financial industry. There is high anticipation for heightened transactional and account security, mainly due to the expansion of new challenges and opportunities due to blockchain and cryptocurrency technology.
Due to the absence of an intermediary, such practice might massively lower transaction fees.
At the same time, cognitive computing will empower digital assistants and apps to be more responsive, making it incredibly easier to manage personal finances and budgeting. Trends like intelligent machines that can plan and execute mundane tasks like bill payment and tax filing will rise.
We can also expect to see a higher quality of customer care due to the emergence of advanced VR systems, natural-language processing, and learning from past experiences.
Comprehensive and precise due diligence tests and KYC reporting would also lead to better client quality and lower defaults and frauds.
Conclusion: Intelligent Adoption Is the Key
It is evident that AI in finance has multiple benefits, and we cannot ignore its existence. Artificial Intelligence is altering how financial institutions generate and utilize data analytics. This specialized technology can also help to customize investments based on individual goals.
As of 2020, 52% of financial service providers have implemented AI-enabled products and processes.23 However, many financial service providers are cautious about the time and cost commitment required to implement AI in digital financial services. However, they would soon realize the potential for these innovative technologies and the return on investment they offer in the long run.
The key personnel spearheading the AI-driven growth trajectory will design, create, and implement FinTech solutions powered by innovative technologies. A combination of funding, technical expertise, domain proficiency, and awareness among senior management will be instrumental in the long-term AI strategy. The companies that will attract multidisciplinary teams with profound experience in AI and financial services have maximum chances of success.
Sources: 1,2,3,4,5,6,7,8,9,10,11,12,13,14,15,16,17,18,19,20,21,22,23,24